Using AI for Financial Analysis: From Data Insights to Decision-Making
Updated on
By Ringy
Table of Contents
Table of Contents
If you're a financial professional, you've likely heard the news about AI for financial analysis—but what does that really mean for you?
In simple terms, it's like having a supercharged assistant who's always on point, never tired, and can crunch numbers faster than you can say "quarterly forecast."
Let's face it—manually sifting through mountains of data is no one's idea of a good time. That's where AI financial analysis comes in.
AI for financial analysis leverages machine learning, natural language processing (NLP), and predictive analytics to transform raw data into actionable insights, helping you make better financial decisions in real-time.
In this article, we'll explore how AI is revolutionizing financial analysis, including practical applications, key benefits, tools you can use, and potential challenges.
How to Use AI for Financial Analysis
Using AI for financial analysis isn't a one-size-fits-all solution, but rather a toolkit of options.
Here's how you can make it work for you.
Collecting and Preparing Financial Data for AI Processing
Before you hand over the data to your shiny new AI for sales assistant, it's important to clean and structure that data. Imagine trying to organize your closet but finding it filled with a mess of mismatched socks and scattered shirts.
Yeah, that's what AI in financial analysis software sees when it tries to process unorganized data.
To get started with the AI financial analyst process, you'll need to make sure your financial data is ready to be processed. That means gathering data from all relevant sources—be it transactional records, income statements, or other financial metrics—and ensuring it's consistent, accurate, and complete.
Gather data from all relevant sources, including:
- Financial statements
- Stock market data
- Transactional records
Make sure it's consistent, complete, and free from errors. Once your data is tidied up, AI for financial analysis can work its magic.
After your data is cleaned up, the next step is integrating it into AI systems for processing. This allows AI tools to better analyze the data, identify trends, and produce insights that are ready for decision-making.
Leveraging AI Tools for Predictive Modeling and Trend Analysis
Now that your data is in shape, it's time to let AI do what it does best: predict the future. By applying machine learning algorithms to your data, AI can identify trends, forecast market behavior, and even alert you to potential financial risks.
For example, if you're working in stock market analysis, AI can examine historical data and uncover hidden patterns to predict how a stock or sector might perform in the future. This gives you a competitive edge, helping you make more informed investment decisions.
Real-Life Example: Want to predict how a stock will perform next quarter? Feed historical data into an AI model, and voilà—AI tells you its prediction based on patterns it's spotted in similar situations.
AI can also analyze vast amounts of financial data in real-time, enabling you to detect shifts in market sentiment, fluctuations in currency exchange rates, or sudden changes in commodity prices—without ever breaking a sweat.
Automating Routine Tasks Like Data Entry and Financial Reporting
Let's face it—managing data entry and generating reports are tasks that no one really enjoys. And with AI, you don't have to! With the right tools, AI can automate these boring but essential tasks, saving you hours each week. It's like having an assistant who's on the clock 24/7, with no coffee breaks required.
Imagine an AI tool that can automatically generate monthly performance reports or forecast financial outcomes based on the latest data. With these AI-powered systems in place, you're free to focus on the higher-level strategy and decision-making, while AI handles the grunt work.
Real-Time Financial Reporting
You know what they say: "Time is money." With AI, you can have real-time financial reporting right at your fingertips. This means you're never more than a few clicks away from up-to-the-minute financial data, whether it's:
- Tracking revenue
- Analyzing expenses
- Measuring KPIs
One of AI's most valuable features is its ability to instantly process and report data in real-time. Whether you're monitoring the performance of a financial portfolio, analyzing quarterly earnings, or gauging overall market sentiment, AI ensures that your reports are always up to date.
Benefits of Using AI for Financial Analysis
Alright, you're convinced that AI can handle your data, but what's in it for you?
Let's talk about the benefits—spoiler alert: they're pretty impressive.
Increased Efficiency
Instead of spending your day clicking through endless spreadsheets, AI helps you get things done faster. Tasks that would take hours can now be completed in minutes.
And let's be honest, who doesn't want more time to focus on strategic decisions instead of getting bogged down by manual data entry?
Not only that, but AI tools and CRM for financial advisors can analyze data faster, and with better insights, than any human can. This means your workflows are streamlined, you spend less time on repetitive tasks, and more time on the tasks that matter—like building customer relationships and closing deals.
Better Accuracy and Reduced Human Error
Remember that one time you mixed up the numbers in a financial report? Yeah, AI and CRM in financial services don't do that.
By automating calculations and predictive models, AI ensures greater accuracy in your:
- Forecasts
- Assessments
- Reports
Since AI systems can handle a massive amount of data without fatigue or error, you can rely on them to deliver precise insights. The more accurate your data, the better your financial decisions will be, and the more confident you can be in your predictions.
Real-Time Financial Reporting
When you need data in real-time, financial analysis AI technology has got your back. Whether you're tracking your portfolio or analyzing quarterly results, AI gives you immediate access to the data you need. Forget about waiting for reports to come in; AI keeps you updated 24/7.
Real-time financial reporting means that you can make adjustments and improvements to your strategy as soon as new data comes in.
Instead of waiting for a monthly report, you can act immediately on fresh data from your CRM system for financial advisors, which is key to staying competitive in today's cutthroat marketplace.
AI-Driven Tools and Technologies in Financial Analysis
AI for financial analysis is like a toolbox with tons of cool gadgets.
Let's break down some of the best tools and technologies available:
Financial AI Calculators
Financial calculator AI software can do everything from tax calculations to budget forecasting. These tools use advanced algorithms to make calculations faster and more accurately than any human could.
AI calculators help eliminate human error, giving you reliable results. They can perform a range of tasks, from calculating net present value (NPV) to estimating future cash flows. With such efficiency, these tools save you hours of manual work.
Example: Let's say you need to calculate potential investment returns for a client. An AI financial calculator can analyze the data, run multiple scenarios, and present the results—all in a matter of seconds.
Machine Learning Platforms for Predictive Insights
Machine learning platforms are like AI's crystal ball.
They help predict everything from stock prices to market trends based on historical data. Imagine having an AI that analyzes the market 24/7, spotting patterns, and making predictions without needing to take a lunch break.
Machine learning platforms are valuable for more than just forecasting stock prices. They can predict loan default risks, assess portfolio performance, and even analyze customer behavior patterns to help financial professionals offer more personalized services.
Natural Language Processing Tools for Analyzing Financial Reports and News
NLP tools let AI read and understand financial reports, earnings calls, and even market news.
Here's a basic look at NLP tools and use cases:
NLP Tool |
Use Case |
Sentiment Analysis |
Detects overall sentiment in market news and reports (positive/negative/neutral). |
Automated Summaries |
Summarizes lengthy financial reports, making them easier to digest. |
NLP can process vast amounts of text from news articles, earnings reports, and press releases, providing you with quick, actionable insights. This helps you stay ahead of the curve in an industry that's constantly evolving.
Choosing the Right AI Tools for Your Financial Needs
With so many options out there, how do you pick the right AI tools?
Here's a quick checklist to help:
- What's your data size? If you're dealing with large datasets, machine-learning platforms may be your best bet.
- What's your budget? Some AI tools are more affordable than others, but they may require additional customization.
- What's the problem you're solving? Whether it's forecasting, risk analysis, or reporting, choose a tool that's built to solve your specific needs.
Consider factors such as ease of integration, accuracy, scalability, and cost. For example, tools like Ringy not only integrate seamlessly with existing financial workflows but also offer tailored analytics that fits your specific industry needs.
Challenges and Ethical Considerations
While AI promises to revolutionize financial analysis, it also presents significant challenges and ethical considerations that must be addressed for successful implementation. As the adoption of AI continues to rise, particularly in financial sectors, the potential for great efficiencies, enhanced decision-making, and predictive insights becomes more evident.
However, these advantages do not come without their complications.
Common Challenges
To better grasp these common challenges, let's break them down in a structured way.
The table below highlights key difficulties faced by financial institutions when adopting AI for financial analysis, and we'll dive deeper into each issue.
Challenge |
Description |
Impact on AI Adoption |
Potential Solutions |
Data Quality and Integration |
Poor-quality data, including inaccuracies and inconsistencies, can lead to unreliable AI outcomes. |
AI models depend on accurate, complete, and clean data to function effectively. If the data used is flawed, predictions and decisions made by AI will be compromised. |
Establish robust data governance, implement data cleansing tools, and ensure systems are compatible with AI. |
Bias and Fairness |
AI models can perpetuate biases found in historical data, leading to discriminatory practices. |
AI's reliance on historical data can reinforce existing inequalities, impacting decisions like loan approvals or credit assessments, potentially excluding vulnerable groups. |
Regular audits for bias, diverse and representative datasets, and the use of Explainable AI (XAI). |
Compliance |
Financial regulations are complex, and AI must adhere to industry standards and laws governing data usage and decision-making. |
Ensuring AI systems comply with laws such as GDPR, the Dodd-Frank Act, or other industry regulations is challenging but necessary to avoid legal repercussions and ensure trust. |
Stay updated on regulatory changes, adopt AI frameworks that promote transparency, and implement compliance audits. |
Lack of Skilled Talent |
There's a shortage of data scientists and AI professionals who can implement and manage AI systems in financial settings. |
Institutions struggle to build AI expertise in-house, limiting the successful deployment of AI systems and their ability to adapt to new technologies. |
Invest in training programs, hire AI specialists, or partner with external consultants to fill talent gaps. |
Integration with Legacy Systems |
Financial institutions often rely on outdated technology that may not be compatible with AI solutions. |
Integrating AI into legacy systems can be resource-intensive and time-consuming, delaying adoption and increasing costs. |
Modernize IT infrastructure, employ middleware solutions, or transition gradually to cloud-based AI solutions. |
As you can see, the challenges of AI adoption in financial analysis are multifaceted. From ensuring data quality to navigating regulatory landscapes, each obstacle demands thoughtful consideration and tailored solutions.
The solutions listed in the table are just starting points, but institutions should work diligently to overcome these barriers, relying on strong data governance practices, fair and unbiased AI development, and an ongoing commitment to staying in compliance with industry regulations.
Ethical Considerations in AI-Driven Financial Analysis
Without oversight, AI can perpetuate biases, lack transparency, or make decisions that miss important human judgment. Institutions must act proactively to ensure AI systems produce ethical outcomes.
Below, we explore key ethical considerations in financial AI.
Transparency
Transparency is critical when using AI in financial services. Many AI systems operate as "black boxes," making it hard for users to understand how decisions are made. Clients must trust that decisions, such as loan approvals or investment choices, are based on understandable and sound reasoning.
To enhance transparency, institutions can adopt Explainable AI (XAI), which clarifies how AI systems reach decisions.
Fairness
AI can unintentionally perpetuate biases, especially if it's trained on data that reflects historical inequalities. This can lead to biased outcomes, such as prioritizing certain demographics while excluding others.
To ensure fairness, institutions should:
- Use diverse datasets to prevent discrimination.
- Audit AI systems regularly to identify and address biases.
- Include diverse teams in AI development to spot potential biases early.
- Seek third-party audits to verify fairness and transparency.
Focusing on fairness ensures that AI systems contribute to an equitable financial environment.
Accountability
While AI excels at processing data quickly, human oversight remains essential, especially in high-stakes financial decisions. Without human judgment, AI can make errors or fail to consider broader socio-economic factors.
Financial institutions should ensure AI remains a tool for human decision-making. This includes:
- Maintaining human oversight over AI-driven decisions.
- Implementing accountability structures to define who is responsible for errors.
- Establishing feedback loops that allow humans to intervene and adjust AI recommendations when necessary.
By keeping human oversight integral, financial institutions can ensure that AI decisions remain accurate and ethically sound.
Final Thoughts - AI for Financial Analysis
AI CRM software is transforming financial analysis by offering faster, more accurate insights, automating tedious tasks, and helping financial professionals make data-driven decisions in real time. From predictive modeling to real-time reporting, AI tools are designed to streamline workflows and improve efficiency.
However, it's crucial to address challenges like data quality, bias, and compliance to ensure ethical and effective implementation.
Ready to harness the power of AI in your financial operations?
Discover how Ringy's CRM sales software and AI tools can help you stay ahead of the curve.
Request a demo today!
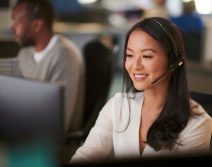
Skyrocket your sales with the CRM that does it all.
Calling? Check. SMS? Check. Automation and AI? Check. Effortlessly keep in touch with your customers and boost your revenue without limits.
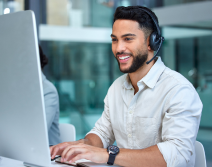
Take your sales to new heights with Ringy.
Sales in a slump? Ringy gives you the tools and flexibility you need to capture leads, engage with them, and turn them into customers.
Subscribe to Our Blog
Enter your email to get the latest updates sent straight to your inbox!